Presse en ligne
Elaborez le maillage de votre site et exploitez votre patrimoine éditorial
Créez un maillage optimal pour votre site en construisant des liens pertinents entre vos articles grâce à la suggestion de contenu similaire et à la détection de mots-clés fournis par Semantic Platform
Exploitez votre patrimoine éditorial pour proposer plus de contenus pertinents à vos lecteurs, en réalignant vos archives sur la taxonomie actuelle du site grâce à la classification automatique
Affinez votre référencement naturel et transformez
vos lecteurs occasionnels en abonnés fidèles
Ils utilisent nos solutions
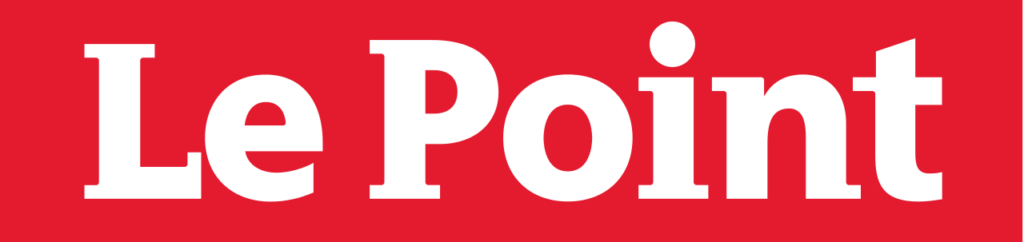


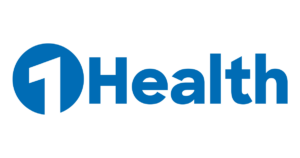
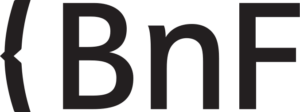
Voyez comment le site Le Point a mesuré l’impact de Semantic Platform sur son audience
édition
Innovez dans la production éditoriale et la diffusion de vos contenus structurés
Produisez plus et mieux avec nos outils novateurs sur mesure, ergonomiques et connectés à vos différents flux
Créez un thésaurus métier pour organiser vos contenus. Découpez, agrégez, étoffez vos contenus pour créer de nouvelles offres
Publiez efficacement les contenus de votre base éditoriale vers votre site web, concevez un portail de contenus fonctionnel
Fidélisez vos clients, optimisez votre production
et composez des offres éditoriales sur mesure
Ils utilisent nos solutions
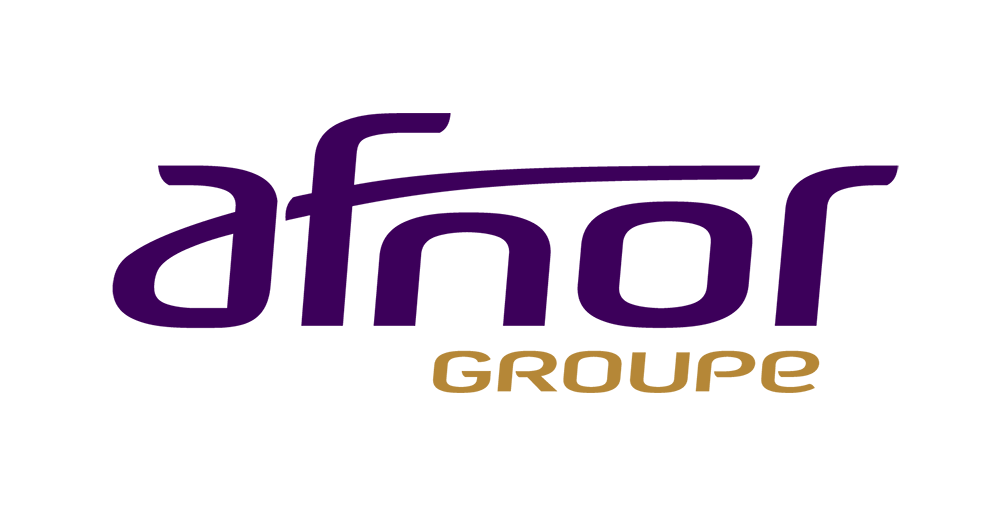
Voyez l’outil novateur créé sur mesure pour la production éditoriale structurée de Lextenso
Actualités
Éthique et IA chez ContentSide
À l’heure où les débats s’enchaînent à propos de l’intelligence artificielle dans le journalisme, ContentSide s’engage pour une utilisation raisonnée, durable et éthique de sa solution Semantic Platform.
ContentSide affirme sa position et adhère pleinement à la Charte de Paris. Arnaud Dumont, le Directeur produit de Semantic Platform, développe plus en détails sa vision dans cet article.